Doing Qualitative Data Analysis in 2025
Qualitative research is undergoing a profound transformation. From initial study planning and participant recruitment, to interviews, transcription, data analysis, and final reporting, each stage of the research workflow is being reshaped by AI-powered tools. The backbone of qualitative research lies in the analysis stage—where researchers code and theme transcripts to uncover insights. This is the most intellectually demanding and time-consuming phase, traditionally done using CAQDAS tools like NVivo, and more recently, with the help of AI-driven platforms such as QualBot. We provide below a detailed comparison between traditional software and AI-native tools, exploring their accuracy, reliability, speed, and market adoption. We also discuss how hybrid human-AI workflows are becoming the future of qualitative research.
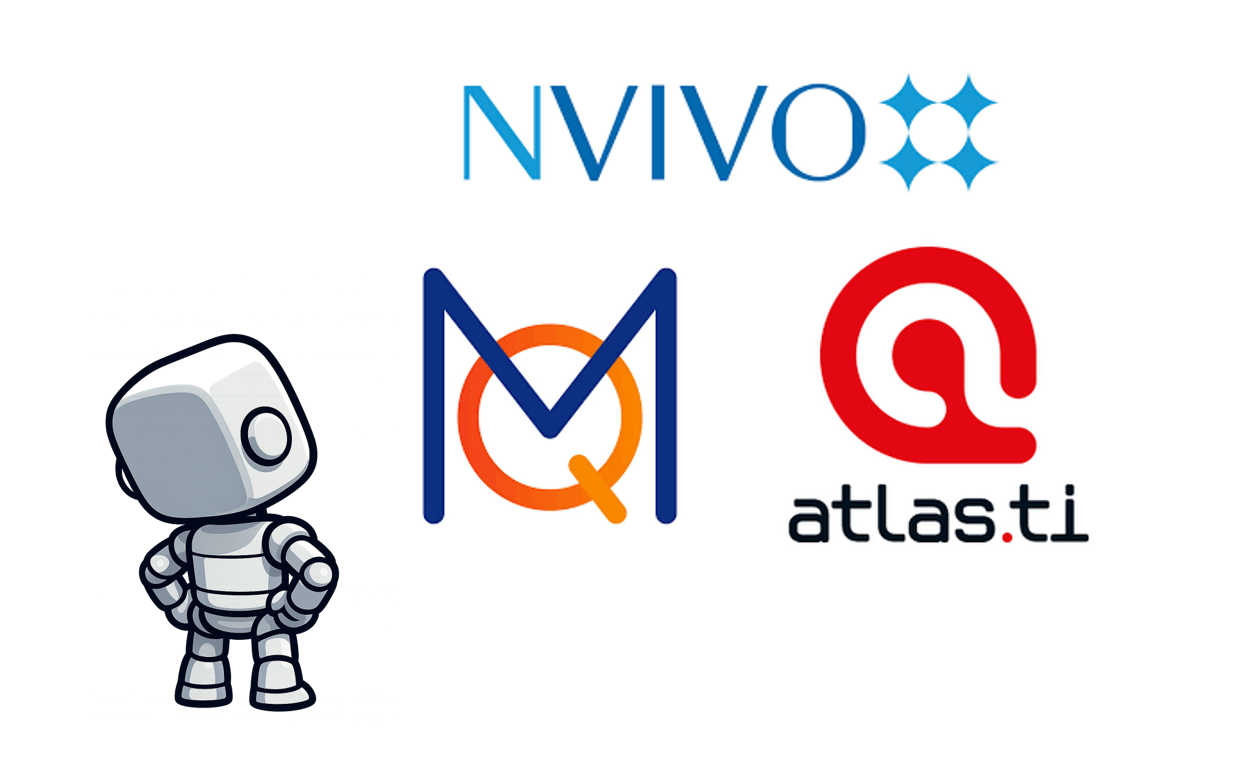
Traditional Tools: NVivo and the CAQDAS Ecosystem
For decades, Computer-Assisted Qualitative Data Analysis Software (CAQDAS) tools like NVivo, ATLAS.ti, and MAXQDA have helped researchers manage large volumes of text data. These tools support manual coding, annotation, and theme-building, offering powerful retrieval, visualization, and querying capabilities. NVivo, often considered the industry standard, allows researchers to:
- Assign codes manually to text segments
- Conduct word frequency and sentiment queries
- Create mind maps and network diagrams
- Generate visualizations and export coded data
These features make it easier to systematize qualitative analysis. However, the intellectual work remains human-led. The researcher must read every transcript, determine what matters, and decide how to code it.
Strengths: Depth of understanding, researcher control, academic rigor, and traceability.
Limitations: Time-intensive, subjective, difficult to scale for large datasets, steep learning curve.
AI-Powered Tools: QualBot and the New Generation
QualBot represents a new generation of qualitative analysis tools powered by advanced AI. It doesn't just support analysis; it performs it. QualBot:
- Ingests all transcripts and performs thematic coding automatically
- Generates a structured codebook with theme definitions and frequencies
- Pulls verbatim quotes for each theme
- Produces a full draft report, including narrative, visuals, and thematic maps
Unlike LLMs used generically for summarization, QualBot uses proprietary workflows like retrieval augmented generation (RAG) to ground every insight in actual transcript data. This ensures every theme is evidence-based and verifiable.
Strengths: Speed, scalability, comprehensiveness, consistency, multilingual support, and automatic documentation.
Limitations: May miss subtle context, interpretive nuance still benefits from human review, less flexible in exploratory phases.
Feature-by-Feature Comparison: NVivo vs. QualBot
Feature | NVivo | QualBot |
---|---|---|
Methodology | Human-led coding | AI-driven thematic analysis |
Coding | Manual + auto-code (basic) | Fully automatic with hierarchical codebook |
Verbatim Quotes | Manually retrieved | Auto-selected, non-redundant quotes per theme |
Visualizations | Mind maps, word clouds | Sunburst charts showing theme hierarchy and frequency |
Accuracy | High with skilled coder | High recall and grounded outputs with quote validation |
Reliability | Depends on human consistency | Model consistency + audit trail for every theme |
Speed | Days to weeks | Minutes to hours |
Effort | High learning curve and manual work | Easy to use; minimal input required |
Market Adoption | Widely used in academia and NGOs | Emerging; rapidly gaining interest in commercial research |
To stay relevant, traditional platforms have started integrating AI. ATLAS.ti and MAXQDA now include AI assistants that:
- Suggest codes based on text selection
- Summarize transcripts
- Offer auto-coding by intent
These hybrid approaches retain human oversight while reducing repetitive tasks. Still, the user remains in the driver's seat, unlike with QualBot, where the AI drafts a full report.
AI in Reporting: From Insight Drafts to Full Deliverables
Reporting is another area where AI is making strides. Tools like QualBot now produce:
- Complete draft reports with background, methods, findings, and quotes
- Thematic maps and visualizations
- Customizable outputs for different audiences
This saves researchers significant time. Instead of spending days crafting a narrative, they can focus on editing and validating AI-generated drafts.
Other tools like Dovetail, Thematic.ai, and HeyMarvin offer similar summarization and auto-tagging features. AI helps distill feedback into insights quickly, though often the final interpretation still benefits from human expertise.
The Hybrid Approach: Best of Both Worlds
The future of qualitative research likely lies in hybrid workflows:
- AI suggests, humans refine: Tools like MAXQDA AI Assist or QualBot Copilot show this well.
- AI codes at scale, humans interpret: QualBot outputs can be reviewed or exported for further human-led analysis.
- Humans design, AI drafts: Researchers input study objectives; AI generates interview guides or draft reports.
This model maintains depth and rigor while boosting efficiency and scalability. In fast-paced industries like UX or market research, this is especially valuable.
Conclusion: Evolving with AI Without Losing the Soul of Qual
AI-powered tools like QualBot are transforming qualitative research. While traditional CAQDAS tools like NVivo remain essential for depth and academic rigor, AI solutions bring unmatched speed and scale. The ideal workflow may combine both: AI for drafting and organizing, and humans for interpretation and insight.
As these tools evolve, researchers will spend less time on repetitive tasks and more time understanding people's stories—the real goal of qualitative research. Whether you're a seasoned NVivo user or an early adopter of AI platforms, embracing the right balance will ensure your insights remain timely, credible, and impactful.